Python 자동화 스크립트로 일상 업무 효율 2배 높이기 🚀
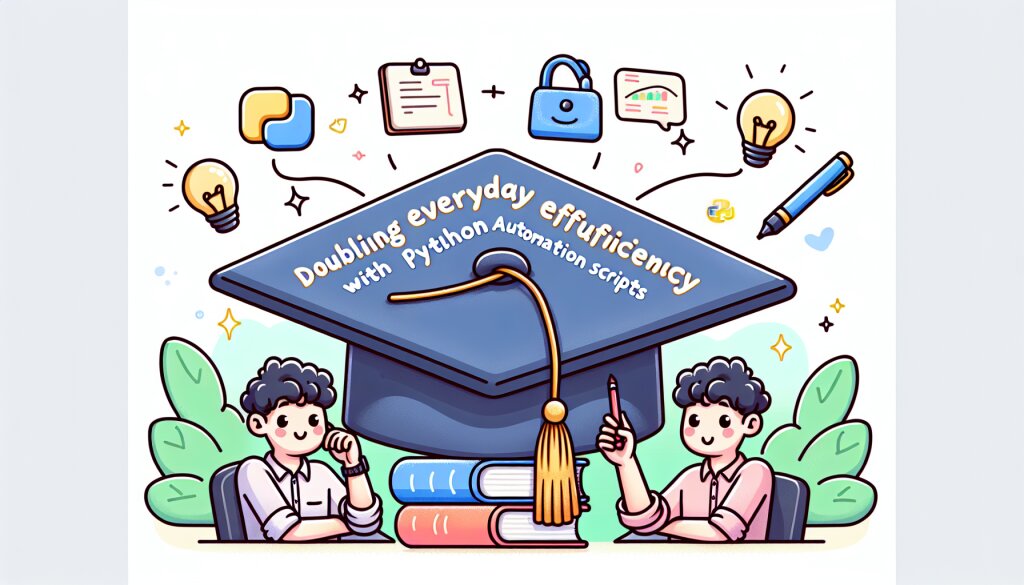
안녕하세요, 여러분! 오늘은 Python을 활용해 일상 업무의 효율성을 획기적으로 높이는 방법에 대해 알아보겠습니다. 🐍✨ 현대 사회에서 시간은 금이라고 하죠. 그만큼 우리의 시간은 소중하고, 효율적으로 사용해야 합니다. 특히 반복적이고 지루한 업무들은 우리의 창의성과 생산성을 저하시키는 주범이 되곤 합니다.
하지만 걱정 마세요! Python이라는 강력한 도구가 여러분의 구원자가 될 거예요. Python은 간결하고 읽기 쉬운 문법, 풍부한 라이브러리, 그리고 뛰어난 확장성으로 유명한 프로그래밍 언어입니다. 이런 특성 덕분에 Python은 자동화 스크립트 작성에 매우 적합하답니다.
이 글에서는 Python을 이용해 다양한 일상 업무를 자동화하는 방법을 상세히 알아볼 예정입니다. 파일 정리부터 데이터 분석, 웹 스크래핑까지! 여러분의 업무 효율을 2배, 아니 그 이상으로 높일 수 있는 방법들을 소개해 드리겠습니다. 😊
Python 초보자부터 중급자까지, 모두가 이해하기 쉽게 설명드릴 테니 걱정 마세요. 여러분도 이 글을 읽고 나면 Python 자동화의 마법에 푹 빠지게 될 거예요!
자, 그럼 Python의 세계로 함께 떠나볼까요? 🚀
1. Python 자동화의 기초 🐍
Python 자동화 스크립트를 작성하기 전에, 먼저 Python의 기본적인 특징과 장점에 대해 알아보겠습니다.
1.1 Python의 특징
- 간결한 문법: Python은 읽기 쉽고 작성하기 쉬운 문법을 가지고 있어요. 중괄호나 세미콜론 같은 복잡한 구문이 필요 없죠.
- 풍부한 라이브러리: Python은 "배터리 포함"이라는 철학을 가지고 있어요. 즉, 기본적으로 많은 유용한 라이브러리들이 포함되어 있답니다.
- 크로스 플랫폼: Windows, macOS, Linux 등 다양한 운영체제에서 동작합니다.
- 인터프리터 언어: 컴파일 과정 없이 바로 실행할 수 있어 빠른 개발이 가능해요.
1.2 Python 설치하기
Python을 사용하기 위해서는 먼저 컴퓨터에 Python을 설치해야 합니다. Python 공식 웹사이트(python.org)에서 최신 버전을 다운로드하여 설치할 수 있어요.
1.3 Python 기본 문법
Python의 기본 문법을 간단히 살펴보겠습니다.
# 변수 선언
name = "Python"
age = 30
# 조건문
if age > 20:
print(f"{name}은 성인입니다.")
else:
print(f"{name}은 미성년자입니다.")
# 반복문
for i in range(5):
print(f"{i}번째 반복")
# 함수 정의
def greet(name):
return f"안녕하세요, {name}님!"
# 함수 호출
message = greet("Python")
print(message)
이러한 기본적인 문법을 이해하고 있다면, 자동화 스크립트를 작성하는 데 큰 도움이 될 거예요.
1.4 Python 자동화의 장점
Python으로 업무를 자동화하면 다음과 같은 장점이 있습니다:
- 시간 절약: 반복적인 작업을 자동화하여 귀중한 시간을 절약할 수 있어요.
- 정확성 향상: 사람의 실수를 줄이고 일관된 결과를 얻을 수 있습니다.
- 확장성: 한 번 작성한 스크립트는 다양한 상황에 맞게 쉽게 수정하고 재사용할 수 있어요.
- 생산성 증가: 단순 작업에서 벗어나 더 창의적이고 가치 있는 일에 집중할 수 있습니다.
이제 Python 자동화의 기초를 알았으니, 다음 섹션에서는 실제로 어떤 업무들을 자동화할 수 있는지 살펴보겠습니다. 🚀
2. 파일 및 폴더 관리 자동화 📁
파일과 폴더를 관리하는 일은 지루하고 시간이 많이 소요되는 작업입니다. 하지만 Python을 사용하면 이러한 작업을 쉽고 빠르게 자동화할 수 있어요. 이 섹션에서는 파일 및 폴더 관리를 자동화하는 다양한 방법을 알아보겠습니다.
2.1 파일 정리하기
먼저, 파일들을 확장자별로 정리하는 스크립트를 만들어 보겠습니다. 이 스크립트는 지정된 폴더 내의 모든 파일을 검사하고, 각 파일의 확장자에 따라 새로운 폴더를 만들어 파일을 이동시킵니다.
import os
import shutil
def organize_files(directory):
for filename in os.listdir(directory):
if os.path.isfile(os.path.join(directory, filename)):
# 파일의 확장자 얻기
file_ext = filename.split('.')[-1]
# 확장자명으로 새 폴더 만들기
new_directory = os.path.join(directory, file_ext)
if not os.path.exists(new_directory):
os.makedirs(new_directory)
# 파일 이동
shutil.move(os.path.join(directory, filename), os.path.join(new_directory, filename))
print("파일 정리가 완료되었습니다!")
# 스크립트 실행
organize_files("/path/to/your/directory")
이 스크립트를 실행하면, 지정된 디렉토리 내의 모든 파일이 확장자별로 분류되어 새로운 폴더로 이동됩니다. 예를 들어, 모든 .pdf 파일은 'pdf' 폴더로, .jpg 파일은 'jpg' 폴더로 이동하게 됩니다.
2.2 대용량 파일 찾기
디스크 공간을 정리할 때, 가장 큰 파일들을 찾아 삭제하거나 이동시키는 것이 효과적입니다. 다음 스크립트는 지정된 디렉토리 내에서 가장 큰 파일들을 찾아 리스트로 보여줍니다.
import os
def find_large_files(directory, top_n=10):
file_sizes = []
for dirpath, dirnames, filenames in os.walk(directory):
for filename in filenames:
filepath = os.path.join(dirpath, filename)
size = os.path.getsize(filepath)
file_sizes.append((filepath, size))
# 파일 크기순으로 정렬
file_sizes.sort(key=lambda x: x[1], reverse=True)
print(f"가장 큰 {top_n}개 파일:")
for filepath, size in file_sizes[:top_n]:
print(f"{filepath}: {size / (1024 * 1024):.2f} MB")
# 스크립트 실행
find_large_files("/path/to/your/directory")
이 스크립트는 지정된 디렉토리와 그 하위 디렉토리를 모두 검사하여 가장 큰 파일 10개를 찾아 보여줍니다. 파일 크기는 MB 단위로 표시됩니다.
2.3 중복 파일 찾기
중복 파일은 불필요하게 디스크 공간을 차지합니다. 다음 스크립트는 중복 파일을 찾아 리스트로 보여줍니다.
import os
import hashlib
def find_duplicate_files(directory):
file_hash_dict = {}
for dirpath, dirnames, filenames in os.walk(directory):
for filename in filenames:
filepath = os.path.join(dirpath, filename)
file_hash = hashlib.md5(open(filepath, 'rb').read()).hexdigest()
if file_hash in file_hash_dict:
file_hash_dict[file_hash].append(filepath)
else:
file_hash_dict[file_hash] = [filepath]
print("중복 파일 목록:")
for file_hash, file_list in file_hash_dict.items():
if len(file_list) > 1:
print(f"\n중복 파일 (MD5: {file_hash}):")
for filepath in file_list:
print(filepath)
# 스크립트 실행
find_duplicate_files("/path/to/your/directory")
이 스크립트는 각 파일의 MD5 해시를 계산하여 동일한 해시를 가진 파일들을 중복 파일로 간주합니다. 중복된 파일들의 경로를 그룹별로 출력합니다.
2.4 파일 이름 일괄 변경
파일 이름을 일괄적으로 변경해야 할 때가 있습니다. 다음 스크립트는 특정 패턴의 파일 이름을 새로운 패턴으로 변경합니다.
import os
import re
def rename_files(directory, pattern, replacement):
for filename in os.listdir(directory):
if os.path.isfile(os.path.join(directory, filename)):
new_filename = re.sub(pattern, replacement, filename)
if new_filename != filename:
os.rename(os.path.join(directory, filename), os.path.join(directory, new_filename))
print(f"Renamed: {filename} -> {new_filename}")
# 스크립트 실행
rename_files("/path/to/your/directory", r"old_pattern", "new_pattern")
이 스크립트는 정규표현식을 사용하여 파일 이름의 특정 패턴을 새로운 패턴으로 변경합니다. 예를 들어, "old_pattern"을 "new_pattern"으로 변경할 수 있습니다.
2.5 파일 백업 자동화
중요한 파일들을 주기적으로 백업하는 것은 매우 중요합니다. 다음 스크립트는 지정된 폴더의 내용을 다른 위치로 백업합니다.
import os
import shutil
import datetime
def backup_files(source_dir, backup_dir):
# 백업 폴더 이름에 날짜 추가
today = datetime.datetime.now().strftime("%Y%m%d")
backup_path = os.path.join(backup_dir, f"backup_{today}")
# 백업 폴더 생성
os.makedirs(backup_path, exist_ok=True)
# 파일 복사
for item in os.listdir(source_dir):
s = os.path.join(source_dir, item)
d = os.path.join(backup_path, item)
if os.path.isdir(s):
shutil.copytree(s, d, symlinks=True)
else:
shutil.copy2(s, d)
print(f"백업이 완료되었습니다: {backup_path}")
# 스크립트 실행
backup_files("/path/to/source/directory", "/path/to/backup/directory")
이 스크립트는 원본 디렉토리의 모든 파일과 폴더를 백업 디렉토리로 복사합니다. 백업 폴더 이름에는 날짜가 포함되어, 여러 번 백업을 수행해도 이전 백업이 덮어씌워지지 않습니다.
이러한 파일 및 폴더 관리 자동화 스크립트들을 활용하면, 수작업으로 하면 몇 시간이 걸릴 수 있는 작업들을 몇 분 만에 완료할 수 있습니다. 이는 업무 효율을 크게 향상시키고, 더 중요한 작업에 집중할 수 있는 시간을 확보해 줍니다.
다음 섹션에서는 데이터 처리와 분석을 자동화하는 방법에 대해 알아보겠습니다. 🚀
3. 데이터 처리 및 분석 자동화 📊
데이터 처리와 분석은 현대 비즈니스에서 매우 중요한 부분입니다. Python은 강력한 데이터 처리 및 분석 도구들을 제공하여, 이러한 작업을 효율적으로 자동화할 수 있게 해줍니다. 이 섹션에서는 Python을 사용한 데이터 처리 및 분석 자동화의 다양한 방법을 살펴보겠습니다.
3.1 CSV 파일 처리
CSV(Comma-Separated Values) 파일은 데이터를 저장하고 교환하는 데 널리 사용되는 형식입니다. Python의 csv 모듈을 사용하면 CSV 파일을 쉽게 읽고 쓸 수 있습니다.
import csv
def process_csv(input_file, output_file):
with open(input_file, 'r') as infile, open(output_file, 'w', newline='') as outfile:
reader = csv.reader(infile)
writer = csv.writer(outfile)
# 헤더 쓰기
header = next(reader)
writer.writerow(header + ['Total'])
# 데이터 처리 및 쓰기
for row in reader:
# 예: 마지막 두 열의 합계 계산
total = sum(map(float, row[-2:]))
writer.writerow(row + [total])
# 스크립트 실행
process_csv('input.csv', 'output.csv')
이 스크립트는 입력 CSV 파일을 읽어 각 행의 마지막 두 열의 합계를 계산하고, 그 결과를 새로운 열에 추가하여 출력 CSV 파일에 저장합니다.
3.2 엑셀 파일 처리
엑셀 파일은 비즈니스 환경에서 매우 흔하게 사용됩니다. Python의 openpyxl 라이브러리를 사용하면 엑셀 파일을 쉽게 다룰 수 있습니다.
from openpyxl import load_workbook, Workbook
def process_excel(input_file, output_file):
# 입력 파일 로드
wb = load_workbook(input_file)
sheet = wb.active
# 새 워크북 생성
new_wb = Workbook()
new_sheet = new_wb.active
# 데이터 처리 및 새 시트에 쓰기
for row in sheet.iter_rows(values_only=True):
# 예: 첫 두 열의 데이터만 복사하고 곱한 값을 추가
new_row = row[:2] + (row[0] * row[1],)
new_sheet.append(new_row)
# 결과 저장
new_wb.save(output_file)
# 스크립트 실행
process_excel('input.xlsx', 'output.xlsx')
이 스크립트는 입력 엑셀 파일의 첫 두 열 데이터를 읽어 새 파일에 복사하고, 두 값의 곱을 세 번째 열에 추가합니다.
3.3 데이터 시각화
데이터 분석 결과를 시각화하는 것은 매우 중요합니다. Python의 matplotlib 라이브러리를 사용하면 다양한 그래프와 차트를 쉽게 만들 수 있습니다.
import matplotlib.pyplot as plt
import numpy as np
def create_chart(data, output_file):
categories = list(data.keys())
values = list(data.values())
plt.figure(figsize=(10, 6))
plt.bar(categories, values)
plt.title('Data Visualization')
plt.xlabel('Categories')
plt.ylabel('Values')
plt.xticks(rotation=45)
plt.tight_layout()
plt.savefig(output_file)
plt.close()
# 샘플 데이터
data = {'A': 10, 'B': 20, 'C': 15, 'D': 25, 'E': 30}
# 스크립트 실행
create_chart(data, 'chart.png')
이 스크립트는 주어진 데이터를 바 차트로 시각화하여 이미지 파일로 저장합니다.
3.4 데이터베이스 작업
많은 기업들이 데이터를 데이터베이스에 저장합니다. Python을 사용하면 데이터베이스 작업을 자동화할 수 있습니다. 여기서는 SQLite를 예로 들어보겠습니다.
import sqlite3
def database_operations():
conn = sqlite3.connect('example.db')
cursor = conn.cursor()
# 테이블 생성
cursor.execute('''CREATE TABLE IF NOT EXISTS users
(id INTEGER PRIMARY KEY, name TEXT, email TEXT)''')
# 데이터 삽입
users = [('John Doe', 'john@example.com'),
('Jane Smith', 'jane@example.com')]
cursor.executemany('INSERT INTO users (name, email) VALUES (?, ?)', users)
# 데이터 조회
cursor.execute('SELECT * FROM users')
for row in cursor.fetchall():
print(row)
conn.commit()
conn.close()
# 스크립트 실행
database_operations()
이 스크립트는 SQLite 데이터베이스를 생성하고, 테이블을 만들고, 데이터를 삽입하고 조회하는 기본적인 데이터베이스 작업을 수행합니다.
3.5 대규모 데이터 처리
대규모 데이터를 처리할 때는 Pandas 라이브러리가 매우 유용합니다. Pandas는 데이터 조작과 분석을 위한 강력한 도구를 제공합니다.
import pandas as pd
def process_large_data(input_file, output_file):
# CSV 파일 읽기
df = pd.read_csv(input_file)
# 데이터 처리
# 예: 'age' 열의 평균으로 결측치 채우기
df['age'].fillna(df['age'].mean(), inplace=True)
# 예: 'salary' 열에 대해 그룹별 평균 계산
grouped = df.groupby('department')['salary'].mean().reset_index()
# 결과 저장
grouped.to_csv(output_file, index=False)
# 스크립트 실행
process_large_data('employees.csv', 'department_salaries.csv')
이 스크립트는 대규모 CSV 파일을 읽어 데이터를 처리하고, 결과를 새 파일로 저장합니다.
데이터 처리와 분석을 자동화함으로써, 우리는 대량의 데이터를 빠르고 정확하게 처리할 수 있습니다. 이는 의사 결정 과정을 개선하고, 비즈니스 인사이트를 얻는 데 큰 도움이 됩니다. 다음 섹션에서는 웹 스크래핑을 통한 데이터 수집 자동화에 대해 알아보겠습니다. 🚀
4. 웹 스크래핑 자동화 🕸️
웹 스크래핑은 웹사이트에서 데이터를 자동으로 추출하는 프로세스입니다. Python은 웹 스크래핑을 위한 강력한 도구들을 제공하여, 온라인 데이터 수집을 효율적으로 자동화할 수 있게 해줍니다. 이 섹션에서는 Python을 사용한 웹 스크래핑 자동화의 다양한 방법을 살펴보겠습니다.
4.1 기본적인 웹 스크래핑
Python의 requests와 BeautifulSoup 라이브러리를 사용하면 간단한 웹 스크래핑을 쉽게 수행할 수 있습니다.
import requests
from bs4 import BeautifulSoup
def scrape_website(url):
# 웹페이지 가져오기
response = requests.get(url)
# BeautifulSoup 객체 생성
soup = BeautifulSoup(response.text, 'html.parser')
# 예: 모든 h1 태그의 텍스트 추출
h1_tags = soup.find_all('h1')
for h1 in h1_tags:
print(h1.text)
# 스크립트 실행
scrape_website('https://example.com')
이 스크립트는 지정된 URL의 웹페이지를 가져와서 모든 h1 태그의 텍스트를 추출합니다.
4.2 동적 웹페이지 스크래핑
JavaScript로 동적으로 콘텐츠를 로드하는 웹사이트의 경우, Selenium을 사용하여 브라우저를 자동화할 수 있습니다.
from selenium import webdriver
from selenium.webdriver.common.by import By
from selenium.webdriver.support.ui import WebDriverWait
from selenium.webdriver.support import expected_conditions as EC
def scrape_dynamic_website(url):
# 웹드라이버 설정
driver = webdriver.Chrome() # Chrome 드라이버 사용
try:
# 웹페이지 로드
driver.get(url)
# 동적으로 로드되는 요소 대기
element = WebDriverWait(driver, 10).until(
EC.presence_of_element_located((By.CLASS_NAME, "dynamic-content"))
)
# 콘텐츠 추출
print(element.text)
finally:
driver.quit()
# 스크립트 실행
scrape_dynamic_website('https://example.com/dynamic-page')
이 스크립트는 동적으로 로드되는 콘텐츠를 기다렸다가 추출합니다.
4.3 대규모 웹 크롤링
여러 페이지를 크롤링해야 할 때는 Scrapy 프레임워크를 사용하면 효율적입니다.
import scrapy
class MySpider(scrapy.Spider):
name = 'myspider'
start_urls = ['https://example.com']
def parse(self, response):
# 페이지에서 데이터 추출
for item in response.css('div.item'):
yield {
'title': item.css('h2::text').get(),
'price': item.css('span.price::text').get(),
}
# 다음 페이지로 이동
next_page = response.css('a.next-page::attr(href)').get()
if next_page is not None:
yield response.follow(next_page, self.parse)
# 스크립트 실행 (터미널에서)
# scrapy runspider myspider.py
이 스크립트는 여러 페이지를 순회하면서 각 페이지에서 항목의 제목과 가격을 추출합니다.
4.4 API를 통한 데이터 수집
많은 웹사이트가 API를 제공합니다. API를 통해 데이터를 수집하면 더 구조화된 형태로 효율적으로 데이터를 얻을 수 있습니다.
import requests
import json
def fetch_api_data(api_url, params=None):
response = requests.get(api_url, params=params)
if response.status_code == 200:
return json.loads(response.text)
else:
print(f"Error: {response.status_code}")
return None
# 스크립트 실행
api_url = 'https://api.example.com/data'
params = {'key': 'your_api_key', 'limit': 10}
data = fetch_api_data(api_url, params)
if data:
for item in data['items']:
print(item['name'], item['value'])
이 스크립트는 API에서 데이터를 가져와 JSON 형식으로 파싱한 후 필요한 정보를 추출합니다.
4.5 웹 스크래핑 윤리와 법적 고려사항
웹 스크래핑을 수행할 때는 다음과 같은 윤리적, 법적 고려사항을 염두에 두어야 합니다:
- 웹사이트의 robots.txt 파일을 확인하고 준수합니다.
- 과도한 요청으로 서버에 부담을 주지 않도록 합니다.
- 저작권 및 개인정보 보호 법규를 준수합니다.
- 가능한 경우 공식 API를 사용합니다.
- 수집한 데이터의 사용 목적을 명확히 합니다.
웹 스크래핑을 자동화함으로써, 우리는 인터넷에서 방대한 양의 데이터를 효율적으로 수집하고 분석할 수 있습니다. 이는 시장 조사, 가격 모니터링, 뉴스 집계 등 다양한 분야에서 활용될 수 있습니다. 다음 섹션에서는 이메일 및 메시징 자동화에 대해 알아보겠습니다. 🚀
5. 이메일 및 메시징 자동화 📧
이메일과 메시징은 현대 비즈니스 커뮤니케이션의 핵심입니다. Python을 사용하면 이러한 커뮤니케이션 프로세스를 효과적으로 자동화할 수 있습니다. 이 섹션에서는 Python을 사용한 이메일 및 메시징 자동화의 다양한 방법을 살펴보겠습니다.
5.1 이메일 전송 자동화
Python의 smtplib 모듈을 사용하면 이메일을 쉽게 보낼 수 있습니다.
import smtplib
from email.mime.text import MIMEText
from email.mime.multipart import MIMEMultipart
def send_email(sender, recipient, subject, body):
# 이메일 설정
message = MIMEMultipart()
message['From'] = sender
message['To'] = recipient
message['Subject'] = subject
# 본문 추가
message.attach(MIMEText(body, 'plain'))
# SMTP 서버 연결 및 이메일 전송
with smtplib.SMTP('smtp.gmail.com', 587) as server:
server.starttls()
server.login(sender, 'your-password')
server.send_message(message)
# 스크립트 실행
send_email('sender@example.com', 'recipient@example.com', 'Test Subject', 'This is a test email.')
이 스크립트는 지정된 수신자에게 이메일을 보냅니다. 실제 사용 시에는 보안을 위해 환경 변수나 설정 파일에서 비밀번호를 가져오는 것이 좋습니다.
5.2 대량 이메일 발송
뉴스레터나 마케팅 캠페인을 위해 대량의 이메일을 보내야 할 때가 있습니다. 다음은 CSV 파일에서 수신자 목록을 읽어 대량 이메일을 보내는 예제입니다.
import csv
from email.mime.text import MIMEText
from email.mime.multipart import MIMEMultipart
import smtplib
def send_bulk_email(sender, subject, body, recipients_file):
with open(recipients_file, 'r') as file:
reader = csv.reader(file)
next(reader) # 헤더 건너뛰기
with smtplib.SMTP('smtp.gmail.com', 587) as server:
server.starttls()
server.login(sender, 'your-password')
for row in reader:
recipient = row[0] # 첫 번째 열이 이메일 주소라고 가정
message = MIMEMultipart()
message['From'] = sender
message['To'] = recipient
message['Subject'] = subject
message.attach(MIMEText(body, 'plain'))
server.send_message(message)
print(f"Email sent to {recipient}")
# 스크립트 실행
send_bulk_email('sender@example.com', 'Bulk Email Subject', 'This is a bulk email.', 'recipients.csv')
이 스크립트는 CSV 파일에서 수신자 목록을 읽어 각 수신자에게 개별적으로 이메일을 보냅니다.
5.3 이메일 자동 응답
특정 이메일 주소로 오는 메일에 자동으로 응답하는 시스템을 만들 수 있습니다. 이는 휴가 중 자동 응답이나 고객 문의에 대한 초기 응답 등에 유용합니다.
import imaplib
import email
import smtplib
from email.mime.text import MIMEText
from email.mime.multipart import MIMEMultipart
def auto_reply():
# IMAP 서버 연결
mail = imaplib.IMAP4_SSL('imap.gmail.com')
mail.login('your-email@gmail.com', 'your-password')
mail.select('inbox')
# 새 이메일 검색
_, search_data = mail.search(None, 'UNSEEN')
for num in search_data[0].split():
_, data = mail.fetch(num, '(RFC822)')
_, bytes_data = data[0]
email_message = email.message_from_bytes(bytes_data)
sender_email = email.utils.parseaddr(email_message['From'])[1]
# 자동 응답 보내기
reply_message = MIMEMultipart()
reply_message['From'] = 'your-email@gmail.com'
reply_message['To'] = sender_email
reply_message['Subject'] = 'Auto Reply: ' + email_message['Subject']
reply_message.attach(MIMEText('This is an automatic response. Thank you for your email.', 'plain'))
with smtplib.SMTP('smtp.gmail.com', 587) as server:
server.starttls()
server.login('your-email@gmail.com', 'your-password')
server.send_message(reply_message)
mail.close()
mail.logout()
# 스크립트 실행
auto_reply()
이 스크립트는 지정된 이메일 계정의 받은 편지함을 확인하고, 새로운 이메일에 대해 자동으로 응답합니다.
5.4 SMS 메시지 전송
Twilio와 같은 서비스를 사용하면 Python으로 SMS 메시지를 보낼 수 있습니다.
from twilio.rest import Client
def send_sms(to_number, message):
# Twilio 계정 정보
account_sid = 'your_account_sid'
auth_token = 'your_auth_token'
from_number = 'your_twilio_number'
client = Client(account_sid, auth_token)
message = client.messages.create(
body=message,
from_=from_number,
to=to_number
)
print(f"Message sent: {message.sid}")
# 스크립트 실행
send_sms('+0', 'This is a test SMS from Python!')
이 스크립트는 Twilio API를 사용하여 지정된 번호로 SMS 메시지를 보냅니다.
5.5 메시징 앱 연동
Slack이나 Discord와 같은 메시징 앱과 연동하여 자동으로 메시지를 보낼 수 있습니다. 다음은 Slack 웹훅을 사용한 예제입니다.
import requests
import json
def send_slack_message(webhook_url, message):
payload = {'text': message}
response = requests.post(
webhook_url,
data=json.dumps(payload),
headers={'Content-Type': 'application/json'}
)
if response.status_code != 200:
raise ValueError(f'Request to Slack returned an error {response.status_code}, the response is:\n{response.text}')
# 스크립트 실행
webhook_url = 'https://hooks.slack.com/services/T00000000/B00000000/XXXXXXXXXXXXXXXXXXXXXXXX'
send_slack_message(webhook_url, 'This is a test message from Python!')
이 스크립트는 지정된 Slack 웹훅 URL로 메시지를 보냅니다.
이메일 및 메시징 자동화를 통해 우리는 효율적이고 일관된 커뮤니케이션을 할 수 있습니다. 이는 고객 서비스 개선, 마케팅 캠페인 관리, 팀 내 협업 강화 등 다양한 분야에서 활용될 수 있습니다. 다음 섹션에서는 보고서 생성 자동화에 대해 알아보겠습니다. 🚀
6. 보고서 생성 자동화 📊
정기적인 보고서 작성은 많은 시간과 노력이 필요한 작업입니다. Python을 사용하면 이러한 보고서 생성 과정을 자동화하여 시간을 절약하고 일관성을 유지할 수 있습니다. 이 섹션에서는 Python을 사용한 보고서 생성 자동화의 다양한 방법을 살펴보겠습니다.
6.1 데이터 수집 및 처리
보고서 생성의 첫 단계는 필요한 데이터를 수집하고 처리하는 것입니다. pandas 라이브러리를 사용하면 이 과정을 효율적으로 수행할 수 있습니다.
import pandas as pd
import numpy as np
def process_data(file_path):
# CSV 파일에서 데이터 읽기
df = pd.read_csv(file_path)
# 데이터 처리
df['total'] = df['quantity'] * df['price']
monthly_sales = df.groupby('month')['total'].sum()
return monthly_sales
# 스크립트 실행
monthly_sales = process_data('sales_data.csv')
print(monthly_sales)
이 스크립트는 CSV 파일에서 판매 데이터를 읽어 월별 총 판매액을 계산합니다.
6.2 차트 및 그래프 생성
데이터를 시각화하면 정보를 더 쉽게 이해할 수 있습니다. matplotlib 라이브러리를 사용하여 차트와 그래프를 생성할 수 있습니다.
import matplotlib.pyplot as plt
def create_chart(data, title, filename):
plt.figure(figsize=(10, 6))
data.plot(kind='bar')
plt.title(title)
plt.xlabel('Month')
plt.ylabel('Sales')
plt.savefig(filename)
plt.close()
# 스크립트 실행
create_chart(monthly_sales, 'Monthly Sales', 'monthly_sales_chart.png')
이 스크립트는 월별 판매 데이터를 바 차트로 시각화하여 이미지 파일로 저장합니다.
6.3 PDF 보고서 생성
최종적으로 데이터와 차트를 포함한 PDF 보고서를 생성할 수 있습니다. reportlab 라이브러리를 사용하면 PDF 문서를 쉽게 만들 수 있습니다.
from reportlab.lib.pagesizes import letter
from reportlab.pdfgen import canvas
from reportlab.lib.utils import ImageReader
def create_pdf_report(data, chart_filename, output_filename):
c = canvas.Canvas(output_filename, pagesize=letter)
width, height = letter
# 제목 추가
c.setFont("Helvetica-Bold", 16)
c.drawString(50, height - 50, "Monthly Sales Report")
# 데이터 테이블 추가
c.setFont("Helvetica", 12)
y = height - 100
for month, sales in data.items():
c.drawString(50, y, f"{month}: ${sales:.2f}")
y -= 20
# 차트 추가
c.drawImage(ImageReader(chart_filename), 50, y - 300, width=400, height=250)
c.save()
# 스크립트 실행
create_pdf_report(monthly_sales, 'monthly_sales_chart.png', 'monthly_sales_report.pdf')
이 스크립트는 월별 판매 데이터와 차트를 포함한 PDF 보고서를 생성합니다.
6.4 자동 이메일 전송
생성된 보고서를 자동으로 이메일로 전송할 수 있습니다. 이전에 살펴본 이메일 전송 코드를 활용할 수 있습니다.
import smtplib
from email.mime.text import MIMEText
from email.mime.multipart import MIMEMultipart
from email.mime.application import MIMEApplication
def send_report_email(sender, recipient, subject, body, attachment_path):
message = MIMEMultipart()
message['From'] = sender
message['To'] = recipient
message['Subject'] = subject
message.attach(MIMEText(body, 'plain'))
with open(attachment_path, 'rb') as f:
attachment = MIMEApplication(f.read(), _subtype="pdf")
attachment.add_header('Content-Disposition', 'attachment', filename='report.pdf')
message.attach(attachment)
with smtplib.SMTP('smtp.gmail.com', 587) as server:
server.starttls()
server.login(sender, 'your-password')
server.send_message(message)
# 스크립트 실행
send_report_email('sender@example.com', 'recipient@example.com', 'Monthly Sales Report',
'Please find attached the monthly sales report.', 'monthly_sales_report.pdf')
이 스크립트는 생성된 PDF 보고서를 첨부하여 이메일로 전송합니다.
6.5 전체 프로세스 자동화
이제 모든 단계를 하나의 함수로 결합하여 전체 보고서 생성 및 전송 프로세스를 자동화할 수 있습니다.
def generate_and_send_report(data_file, recipient):
# 데이터 처리
monthly_sales = process_data(data_file)
# 차트 생성
create_chart(monthly_sales, 'Monthly Sales', 'monthly_sales_chart.png')