Java Stream API로 데이터 처리 최적화하기 🚀
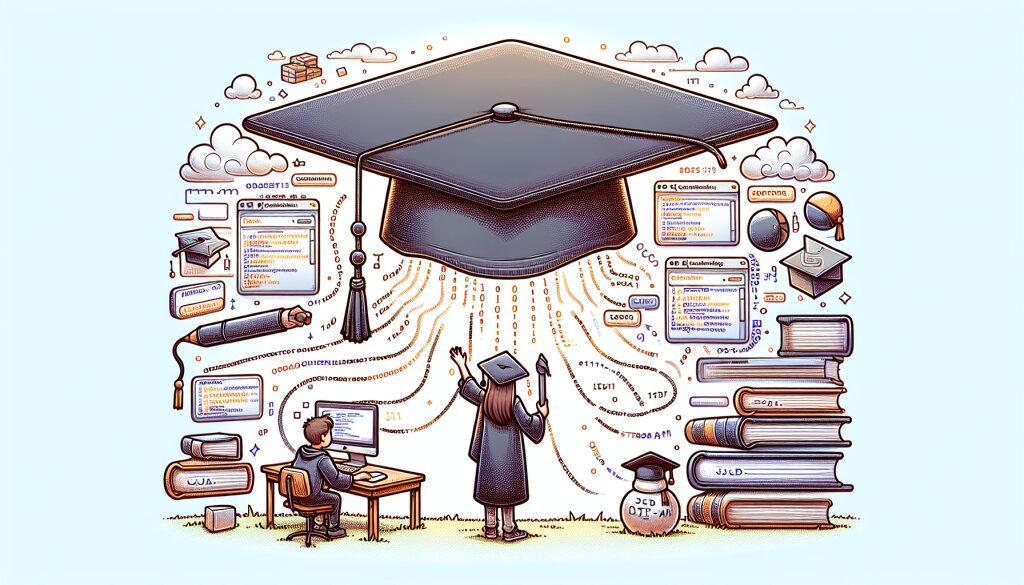
안녕하세요, 재능넷 독자 여러분! 오늘은 Java 개발자들에게 매우 중요한 주제인 'Java Stream API를 활용한 데이터 처리 최적화'에 대해 깊이 있게 알아보겠습니다. 이 글을 통해 여러분은 Java 프로그래밍 실력을 한 단계 더 높일 수 있을 것입니다. 😊
Java Stream API는 Java 8에서 도입된 혁신적인 기능으로, 데이터 처리를 위한 강력하고 유연한 도구입니다. 이를 통해 개발자들은 더 간결하고 효율적인 코드를 작성할 수 있게 되었죠. 특히 대용량 데이터를 다루는 현대 애플리케이션 개발에 있어 Stream API의 중요성은 날로 커지고 있습니다.
이 글에서는 Stream API의 기본 개념부터 시작하여, 고급 기능과 최적화 테크닉까지 상세히 다룰 예정입니다. 재능넷의 '지식인의 숲' 메뉴에 게시되는 이 글을 통해, 여러분의 Java 프로그래밍 실력이 한층 더 발전하기를 희망합니다.
그럼 지금부터 Java Stream API의 세계로 함께 떠나볼까요? 🌊
1. Java Stream API 소개 📚
Java Stream API는 Java 8에서 도입된 혁신적인 기능입니다. 이는 컬렉션을 처리하는 새로운 방식을 제공하며, 함수형 프로그래밍의 장점을 Java에 도입했습니다. Stream API를 사용하면 데이터 소스를 추상화하고, 데이터를 다루는 공통 작업을 표현할 수 있습니다.
1.1 Stream API의 주요 특징
- 선언적 프로그래밍: Stream API를 사용하면 "무엇"을 할지 선언하는 방식으로 프로그래밍할 수 있습니다. 이는 기존의 명령형 프로그래밍과는 다른 접근 방식입니다.
- 함수형 프로그래밍 지원: 람다 표현식과 함께 사용하여 간결하고 표현력 있는 코드를 작성할 수 있습니다.
- 파이프라이닝: 여러 작업을 연결하여 복잡한 데이터 처리 파이프라인을 구성할 수 있습니다.
- 내부 반복: 컬렉션 내부에서 요소를 반복 처리하므로, 개발자가 직접 반복문을 작성할 필요가 없습니다.
- 지연 연산: 최종 연산이 호출되기 전까지는 중간 연산이 실행되지 않아 효율적인 처리가 가능합니다.
1.2 Stream API vs 전통적인 컬렉션 처리
Stream API와 전통적인 컬렉션 처리 방식의 차이를 간단한 예제를 통해 살펴보겠습니다.
위의 예제에서 볼 수 있듯이, Stream API를 사용하면 코드가 더 간결하고 읽기 쉬워집니다. 또한, 각 단계가 명확히 구분되어 있어 코드의 의도를 파악하기 쉽습니다.
1.3 Stream API의 장점
- 코드 간결성: 복잡한 로직을 간결하게 표현할 수 있습니다.
- 가독성 향상: 데이터 처리 과정이 명확히 드러나 코드의 의도를 쉽게 파악할 수 있습니다.
- 유지보수 용이성: 코드 변경이 필요할 때 관련 부분만 수정하면 되므로 유지보수가 쉬워집니다.
- 병렬 처리 지원: 간단한 메서드 호출만으로 멀티코어 프로세서의 힘을 활용할 수 있습니다.
- 최적화된 성능: 내부적으로 최적화된 알고리즘을 사용하여 대량의 데이터를 효율적으로 처리합니다.
이러한 장점들로 인해 Stream API는 현대 Java 프로그래밍에서 필수적인 도구가 되었습니다. 재능넷과 같은 플랫폼에서 Java 개발 관련 지식을 공유할 때, Stream API의 활용은 매우 중요한 주제로 다뤄지고 있죠.
다음 섹션에서는 Stream API의 기본 구조와 주요 연산에 대해 자세히 알아보겠습니다. 🌟
2. Stream API의 기본 구조와 주요 연산 🔧
Stream API를 효과적으로 사용하기 위해서는 그 기본 구조와 주요 연산을 이해하는 것이 중요합니다. 이 섹션에서는 Stream의 생성부터 중간 연산, 최종 연산까지 상세히 살펴보겠습니다.
2.1 Stream의 기본 구조
Stream은 크게 세 부분으로 구성됩니다:
- 데이터 소스: Stream의 대상이 되는 데이터 집합
- 중간 연산: 데이터를 변환하거나 필터링하는 연산들의 체인
- 최종 연산: 결과를 도출하는 연산
2.2 Stream 생성
Stream은 다양한 방법으로 생성할 수 있습니다:
- 컬렉션으로부터 생성:
collection.stream()
- 배열로부터 생성:
Arrays.stream(array)
- 숫자 범위로부터 생성:
IntStream.range(1, 100)
- 파일로부터 생성:
Files.lines(path)
- 직접 생성:
Stream.of("a", "b", "c")
예를 들어, 리스트로부터 Stream을 생성하는 코드는 다음과 같습니다:
List<String> myList = Arrays.asList("a", "b", "c");
Stream<String> myStream = myList.stream();
2.3 중간 연산 (Intermediate Operations)
중간 연산은 Stream을 변환하거나 필터링하는 역할을 합니다. 중간 연산의 특징은 지연 연산(lazy evaluation)이라는 점입니다. 즉, 최종 연산이 호출되기 전까지는 실제로 실행되지 않습니다.
주요 중간 연산들은 다음과 같습니다:
- filter(): 조건에 맞는 요소만 선택
- map(): 각 요소를 변환
- flatMap(): 각 요소를 Stream으로 변환 후 하나의 Stream으로 평면화
- distinct(): 중복 제거
- sorted(): 정렬
- peek(): 각 요소를 순회하며 특정 작업 수행 (주로 디버깅용)
- limit(): 처음 n개의 요소만 선택
- skip(): 처음 n개의 요소를 제외
예를 들어, 문자열 리스트에서 길이가 3 이상인 문자열만 대문자로 변환하는 코드는 다음과 같습니다:
List<String> result = myList.stream()
.filter(s -> s.length() >= 3)
.map(String::toUpperCase)
.collect(Collectors.toList());
2.4 최종 연산 (Terminal Operations)
최종 연산은 Stream의 요소를 소모하여 최종 결과를 도출합니다. 최종 연산이 수행되면 Stream은 소모되어 더 이상 사용할 수 없게 됩니다.
주요 최종 연산들은 다음과 같습니다:
- forEach(): 각 요소에 대해 특정 작업 수행
- collect(): 요소를 수집하여 컬렉션 등의 자료구조로 변환
- reduce(): 요소를 하나로 줄이는 연산 수행
- count(): 요소의 개수 반환
- anyMatch(): 조건을 만족하는 요소가 하나라도 있는지 확인
- allMatch(): 모든 요소가 조건을 만족하는지 확인
- noneMatch(): 모든 요소가 조건을 만족하지 않는지 확인
- findFirst(): 첫 번째 요소 반환
- findAny(): 아무 요소나 반환 (병렬 처리 시 유용)
- min(): 최솟값 요소 반환
- max(): 최댓값 요소 반환
예를 들어, 숫자 리스트의 합계를 구하는 코드는 다음과 같습니다:
List<Integer> numbers = Arrays.asList(1, 2, 3, 4, 5);
int sum = numbers.stream()
.reduce(0, (a, b) -> a + b);
2.5 Stream 연산의 특징
- 파이프라이닝: 대부분의 Stream 연산은 Stream을 반환하므로, 메서드 체이닝을 통해 파이프라인을 구성할 수 있습니다.
- 내부 반복: Stream은 요소들의 내부 반복을 알아서 처리하므로, 개발자는 작업의 로직에만 집중할 수 있습니다.
- 지연 연산: 중간 연산은 최종 연산이 호출될 때까지 실행되지 않습니다. 이를 통해 불필요한 연산을 줄일 수 있습니다.
- 순서: 대부분의 연산은 순서를 유지하지만, 일부 연산(예:
unordered()
)은 순서를 무시할 수 있습니다. - 1회용: Stream은 한 번 사용하면 닫히므로, 재사용할 수 없습니다. 필요하다면 새로운 Stream을 생성해야 합니다.
이러한 Stream API의 기본 구조와 연산들을 이해하면, 복잡한 데이터 처리 작업을 효율적으로 수행할 수 있습니다. 재능넷에서 Java 프로그래밍 관련 지식을 공유할 때, 이러한 Stream API의 기본 개념은 매우 중요한 토픽이 될 것입니다.
다음 섹션에서는 Stream API를 활용한 실전 예제와 최적화 기법에 대해 더 자세히 알아보겠습니다. 🚀
3. Stream API 활용 실전 예제 💼
이제 Stream API의 기본 개념을 이해했으니, 실제 프로그래밍 상황에서 어떻게 활용할 수 있는지 살펴보겠습니다. 다양한 시나리오를 통해 Stream API의 강력함을 경험해 보세요.
3.1 데이터 필터링과 변환
직원 정보를 담은 리스트에서 특정 조건을 만족하는 직원들의 이름을 추출하는 예제를 살펴보겠습니다.
class Employee {
String name;
int age;
String department;
double salary;
// 생성자, getter, setter 생략
}
List<Employee> employees = // 직원 정보 리스트
List<String> highPaidITEmployees = employees.stream()
.filter(e -> e.getDepartment().equals("IT"))
.filter(e -> e.getSalary() > 50000)
.map(Employee::getName)
.collect(Collectors.toList());
이 예제에서는 IT 부서에 근무하며 연봉이 50,000 이상인 직원들의 이름을 추출합니다. filter()
를 통해 조건을 적용하고, map()
을 사용해 Employee 객체에서 이름만 추출합니다.
3.2 데이터 집계
이번에는 부서별 평균 연봉을 계산하는 예제를 살펴보겠습니다.
Map<String, Double> avgSalaryByDept = employees.stream()
.collect(Collectors.groupingBy(
Employee::getDepartment,
Collectors.averagingDouble(Employee::getSalary)
));
이 코드는 groupingBy()
를 사용하여 부서별로 그룹화하고, averagingDouble()
을 통해 각 그룹의 평균 연봉을 계산합니다.
3.3 복잡한 데이터 처리
이제 좀 더 복잡한 데이터 처리 예제를 살펴보겠습니다. 각 부서에서 가장 높은 연봉을 받는 직원을 찾아보겠습니다.
Map<String, Optional<Employee>> highestPaidByDept = employees.stream()
.collect(Collectors.groupingBy(
Employee::getDepartment,
Collectors.maxBy(Comparator.comparingDouble(Employee::getSalary))
));
이 예제에서는 groupingBy()
로 부서별로 그룹화한 후, maxBy()
를 사용하여 각 그룹에서 연봉이 가장 높은 직원을 찾습니다.
3.4 병렬 처리
Stream API의 강력한 기능 중 하나는 병렬 처리입니다. 대량의 데이터를 처리할 때 매우 유용합니다.
long count = employees.parallelStream()
.filter(e -> e.getSalary() > 100000)
.count();
이 코드는 parallelStream()
을 사용하여 병렬로 처리합니다. 연봉이 100,000 이상인 직원의 수를 빠르게 계산할 수 있습니다.
3.5 커스텀 컬렉터 만들기
때로는 기본 제공되는 컬렉터로는 부족할 수 있습니다. 이런 경우 커스텀 컬렉터를 만들어 사용할 수 있습니다.
class EmployeeStats {
int count;
double sumSalary;
double maxSalary;
// 생성자, getter, setter 생략
}
Collector<Employee, EmployeeStats, EmployeeStats> customCollector = Collector.of(
EmployeeStats::new,
(stats, emp) -> {
stats.count++;
stats.sumSalary += emp.getSalary();
stats.maxSalary = Math.max(stats.maxSalary, emp.getSalary());
},
(stats1, stats2) -> {
stats1.count += stats2.count;
stats1.sumSalary += stats2.sumSalary;
stats1.maxSalary = Math.max(stats1.maxSalary, stats2.maxSalary);
return stats1;
}
);
EmployeeStats stats = employees.stream().collect(customCollector);
이 예제에서는 직원 수, 총 연봉, 최대 연봉을 한 번에 계산하는 커스텀 컬렉터를 만들었습니다.
3.6 무한 스트림 활용
Stream API는 무한 스트림도 지원합니다. 이를 활용하여 특정 조건을 만족하는 요소를 찾을 때까지 계속 생성할 수 있습니다.
Random random = new Random();
Stream<Integer> infiniteStream = Stream.generate(() -> random.nextInt(100));
List<Integer> randomNumbers = infiniteStream
.limit(10)
.filter(n -> n % 2 == 0)
.collect(Collectors.toList());
이 예제에서는 무작위 숫자를 생성하는 무한 스트림을 만들고, 그 중 처음 10개의 짝수만을 선택합니다.
3.7 파일 처리
Stream API는 파일 처리에도 매우 유용합니다. 대용량 로그 파일에서 특정 패턴을 찾는 예제를 살펴보겠습니다.
try (Stream<String> lines = Files.lines(Paths.get("large_log_file.txt"))) {
List<String> errorLogs = lines
.filter(line -> line.contains("ERROR"))
.limit(100)
.collect(Collectors.toList());
} catch (IOException e) {
e.printStackTrace();
}
이 코드는 대용량 로그 파일에서 "ERROR"를 포함하는 첫 100개의 라인만을 추출합니다.
3.8 데이터 변환 및 플랫맵
복잡한 객체 구조를 다룰 때 flatMap()
은 매우 유용합니다. 부서별 직원 목록을 평면화하는 예제를 보겠습니다.
class Department {
String name;
List<Employee> employees;
// 생성자, getter, setter 생략
}
List<Department> departments = // 부서 정보 리스트
List<Employee> allEmployees = departments.stream()
.flatMap(dept -> dept.getEmployees().stream())
.collect(Collectors.toList());
이 예제에서는 flatMap()
을 사용하여 각 부서의 직원 리스트를 하나의 평면화된 스트림으로 변환합니다.
3.9 요소 결합
Stream의 요소들을 하나의 결과로 결합하는 reduce()
연산을 살펴보겠습니다.
Optional<Employee> employeeWithHighestSalary = employees.stream()
.reduce((e1, e2) -> e1.getSalary() > e2.getSalary() ? e1 : e2);
employeeWithHighestSalary.ifPresent(e ->
System.out.println("Highest paid employee: " + e.getName()));
이 예제는 전체 직원 중 가장 높은 연봉을 받는 직원을 찾습니다.
3.10 복잡한 정 렬
Stream API를 사용하면 복잡한 정렬 로직도 쉽게 구현할 수 있습니다. 여러 기준으로 정렬하는 예제를 살펴보겠습니다.
List<Employee> sortedEmployees = employees.stream()
.sorted(Comparator
.comparing(Employee::getDepartment)
.thenComparing(Employee::getSalary, Comparator.reverseOrder())
.thenComparing(Employee::getName))
.collect(Collectors.toList());
이 예제에서는 직원들을 부서별로 먼저 정렬하고, 같은 부서 내에서는 연봉 내림차순으로, 연봉이 같다면 이름 알파벳 순으로 정렬합니다.
3.11 통계 계산
Stream API는 기본적인 통계 계산 기능을 제공합니다. 연봉에 대한 다양한 통계를 계산하는 예제를 보겠습니다.
DoubleSummaryStatistics salaryStats = employees.stream()
.mapToDouble(Employee::getSalary)
.summaryStatistics();
System.out.println("Average salary: " + salaryStats.getAverage());
System.out.println("Max salary: " + salaryStats.getMax());
System.out.println("Min salary: " + salaryStats.getMin());
System.out.println("Total salary: " + salaryStats.getSum());
System.out.println("Number of employees: " + salaryStats.getCount());
이 코드는 한 번의 스트림 연산으로 평균, 최대, 최소, 합계, 개수 등의 통계를 모두 계산합니다.
3.12 Optional과 함께 사용
Stream API는 Java 8에서 도입된 Optional 클래스와 잘 어울립니다. null 체크를 우아하게 처리하는 예제를 살펴보겠습니다.
Optional<Employee> oldestEmployee = employees.stream()
.max(Comparator.comparingInt(Employee::getAge));
String oldestEmployeeName = oldestEmployee
.map(Employee::getName)
.orElse("No employee found");
System.out.println("Oldest employee: " + oldestEmployeeName);
이 예제는 가장 나이 많은 직원을 찾고, 그 직원의 이름을 안전하게 추출합니다.
3.13 중첩된 컬렉션 처리
복잡한 데이터 구조에서 특정 정보를 추출하는 예제를 살펴보겠습니다.
class Project {
String name;
List<Task> tasks;
// 생성자, getter, setter 생략
}
class Task {
String description;
List<String> tags;
// 생성자, getter, setter 생략
}
List<Project> projects = // 프로젝트 리스트
Set<String> allTags = projects.stream()
.flatMap(project -> project.getTasks().stream())
.flatMap(task -> task.getTags().stream())
.collect(Collectors.toSet());
이 예제는 모든 프로젝트의 모든 태스크에 있는 모든 태그를 중복 없이 수집합니다.
3.14 조건부 스트림 처리
특정 조건에 따라 다른 스트림 처리를 하고 싶을 때 사용할 수 있는 패턴입니다.
boolean includeInterns = true;
Stream<Employee> employeeStream = employees.stream();
if (includeInterns) {
employeeStream = Stream.concat(employeeStream, interns.stream());
}
List<String> allNames = employeeStream
.map(Employee::getName)
.collect(Collectors.toList());
이 예제는 조건에 따라 인턴을 포함할지 결정하고, 모든 직원의 이름을 수집합니다.
3.15 스트림 디버깅
복잡한 스트림 연산을 디버깅할 때 peek()
메서드를 활용할 수 있습니다.
List<String> debuggedList = employees.stream()
.filter(e -> e.getSalary() > 50000)
.peek(e -> System.out.println("After salary filter: " + e.getName()))
.filter(e -> e.getAge() > 30)
.peek(e -> System.out.println("After age filter: " + e.getName()))
.map(Employee::getName)
.collect(Collectors.toList());
이 예제는 각 필터 단계 후의 결과를 출력하여 스트림 처리 과정을 추적할 수 있게 합니다.
이러한 다양한 예제들을 통해 Stream API의 강력함과 유연성을 확인할 수 있습니다. 재능넷에서 Java 프로그래밍 지식을 공유할 때, 이런 실전적인 예제들은 독자들의 이해를 크게 도울 것입니다.
다음 섹션에서는 Stream API를 사용할 때의 주의사항과 최적화 기법에 대해 알아보겠습니다. 🚀
4. Stream API 사용 시 주의사항 및 최적화 기법 🛠️
Stream API는 강력한 도구이지만, 효과적으로 사용하기 위해서는 몇 가지 주의사항과 최적화 기법을 알아야 합니다. 이 섹션에서는 Stream API를 사용할 때 주의해야 할 점들과 성능을 향상시킬 수 있는 방법들을 살펴보겠습니다.
4.1 무분별한 병렬 스트림 사용 주의
병렬 스트림(parallelStream()
)은 멀티코어 환경에서 성능을 향상시킬 수 있지만, 항상 좋은 것은 아닙니다.
- 작은 데이터셋에서는 오히려 성능이 저하될 수 있습니다.
- 병렬화 과정의 오버헤드가 실제 처리 시간보다 클 수 있습니다.
- 공유 상태를 수정하는 작업에서는 동기화 문제가 발생할 수 있습니다.
예시:
// 병렬 스트림이 항상 좋은 것은 아닙니다
List<Integer> numbers = Arrays.asList(1, 2, 3, 4, 5);
int sum = numbers.parallelStream()
.reduce(0, (a, b) -> a + b); // 작은 리스트에서는 오히려 느릴 수 있습니다
4.2 상태 변경 주의
Stream 연산 중에 외부 상태를 변경하는 것은 위험할 수 있습니다. 특히 병렬 스트림에서는 예측할 수 없는 결과를 초래할 수 있습니다.
// 잘못된 예시
List<Integer> numbers = new ArrayList<>();
IntStream.range(0, 1000)
.parallel()
.forEach(i -> numbers.add(i)); // 동시성 문제 발생 가능
// 올바른 예시
List<Integer> numbers = IntStream.range(0, 1000)
.parallel()
.boxed()
.collect(Collectors.toList());
4.3 무한 스트림 사용 시 주의
무한 스트림을 사용할 때는 반드시 제한을 두어야 합니다. 그렇지 않으면 프로그램이 무한 루프에 빠질 수 있습니다.
// 잘못된 예시
Stream.iterate(0, i -> i + 1)
.forEach(System.out::println); // 무한 루프
// 올바른 예시
Stream.iterate(0, i -> i + 1)
.limit(100)
.forEach(System.out::println);
4.4 스트림 재사용 금지
스트림은 한 번 사용하면 닫히므로 재사용할 수 없습니다. 재사용 시 IllegalStateException
이 발생합니다.
Stream<String> stream = list.stream();
long count = stream.count();
List<String> collected = stream.collect(Collectors.toList()); // 예외 발생
4.5 short-circuiting 연산 활용
무한 스트림이나 대용량 데이터를 다룰 때는 short-circuiting 연산(limit()
, findFirst()
, anyMatch()
등)을 활용하여 불필요한 연산을 줄일 수 있습니다.
boolean hasNegativeNumber = numbers.stream()
.anyMatch(n -> n < 0); // 음수를 찾으면 즉시 종료
4.6 적절한 데이터 구조 선택
스트림 연산의 효율성은 기반 데이터 구조에 따라 달라질 수 있습니다. 예를 들어, ArrayList
는 순차 접근에 효율적이지만, LinkedList
는 그렇지 않습니다.
// ArrayList가 더 효율적
List<Integer> arrayList = new ArrayList<>(numbers);
int sum = arrayList.stream().reduce(0, Integer::sum);
// LinkedList는 순차 접근에 비효율적
List<Integer> linkedList = new LinkedList<>(numbers);
int sum = linkedList.stream().reduce(0, Integer::sum);
4.7 기본형 특화 스트림 사용
기본 타입(int, long, double)을 다룰 때는 기본형 특화 스트림(IntStream
, LongStream
, DoubleStream
)을 사용하면 성능이 향상됩니다.
// 박싱/언박싱 오버헤드 발생
Stream<Integer> boxedStream = Stream.of(1, 2, 3, 4, 5);
int sum = boxedStream.reduce(0, Integer::sum);
// 기본형 특화 스트림 사용으로 성능 향상
IntStream intStream = IntStream.of(1, 2, 3, 4, 5);
int sum = intStream.sum();
4.8 효율적인 필터링
여러 필터를 적용할 때는 계산 비용이 적은 필터를 먼저 적용하는 것이 효율적입니다.
employees.stream()
.filter(e -> e.getAge() > 30) // 간단한 조건 먼저
.filter(e -> e.getSalary() > 50000) // 복잡한 조건 나중에
.collect(Collectors.toList());
4.9 중간 연산 결과 확인
복잡한 스트림 파이프라인을 디버깅할 때는 peek()
메서드를 사용하여 중간 결과를 확인할 수 있습니다.
List<String> result = employees.stream()
.filter(e -> e.getSalary() > 50000)
.peek(e -> System.out.println("Filtered: " + e.getName()))
.map(Employee::getName)
.peek(name -> System.out.println("Mapped: " + name))
.collect(Collectors.toList());
4.10 컬렉터 최적화
적절한 컬렉터를 선택하면 성능을 향상시킬 수 있습니다. 예를 들어, 결과가 Set이어도 될 때는 toSet()
을 사용하는 것이 toList()
보다 효율적일 수 있습니다.
// List로 수집 (중복 허용)
List<String> nameList = employees.stream()
.map(Employee::getName)
.collect(Collectors.toList());
// Set으로 수집 (중복 제거, 더 효율적일 수 있음)
Set<String> nameSet = employees.stream()
.map(Employee::getName)
.collect(Collectors.toSet());
4.11 지연 연산 활용
Stream API의 지연 연산 특성을 이해하고 활용하면 성능을 크게 향상시킬 수 있습니다.
Optional<Employee> firstHighPaidEmployee = employees.stream()
.filter(e -> {
System.out.println("Filtering " + e.getName());
return e.getSalary() > 100000;
})
.findFirst();
// 조건을 만족하는 첫 번째 요소를 찾으면 나머지 요소는 처리하지 않습니다
4.12 병렬 스트림 사용 시 고려사항
병렬 스트림을 효과적으로 사용하기 위해서는 다음 사항들을 고려해야 합니다:
- 데이터 소스가 쉽게 분할 가능한지 (예: ArrayList는 분할이 쉽지만, LinkedList는 어려움)
- 작업이 독립적이고 상태를 공유하지 않는지
- 결과 조합이 비용이 많이 드는 작업인지
// 병렬 스트림이 효과적인 경우
long sum = IntStream.rangeClosed(1, 1_000_000)
.parallel()
.sum();
// 병렬 스트림이 비효율적일 수 있는 경우
List<String> words = // 큰 단어 리스트
String concatenated = words.parallelStream()
.reduce("", (a, b) -> a + b); // 문자열 연결은 병렬화에 적합하지 않음
4.13 복잡한 집계 연산 최적화
복잡한 집계 연산을 수행할 때는 커스텀 컬렉터를 사용하거나, 여러 연산을 하나로 결합하는 것이 효율적일 수 있습니다.
// 여러 통계를 한 번에 계산
DoubleSummaryStatistics stats = employees.stream()
.collect(Collectors.summarizingDouble(Employee::getSalary));
System.out.println("Average: " + stats.getAverage());
System.out.println("Max: " + stats.getMax());
System.out.println("Min: " + stats.getMin());
4.14 메모리 사용 최적화
대용량 데이터를 처리할 때는 메모리 사용을 최적화해야 합니다. 필요한 경우 스트림을 청크로 나누어 처리하거나, 외부 저장소를 활용할 수 있습니다.
// 파일을 라인 단위로 처리하여 메모리 사용 최적화
try (Stream<String> lines = Files.lines(Paths.get("huge_file.txt"))) {
lines.filter(line -> line.contains("important"))
.forEach(System.out::println);
} catch (IOException e) {
e.printStackTrace();
}
이러한 주의사항과 최적화 기법들을 숙지하고 적용하면, Stream API를 더욱 효과적으로 사용할 수 있습니다. 재능넷에서 Java 프로그래밍 지식을 공유할 때, 이러한 실용적인 팁들은 독자들에게 큰 도움이 될 것입니다.
다음 섹션에서는 Stream API의 실제 활용 사례와 함께, 더 깊이 있는 최적화 기법에 대해 알아보겠습니다. 🚀
5. Stream API의 실제 활용 사례 및 고급 최적화 기법 🔍
이 섹션에서는 Stream API의 실제 활용 사례를 살펴보고, 더 깊이 있는 최적화 기법에 대해 알아보겠습니다. 실제 프로젝트에서 Stream API를 어떻게 활용할 수 있는지, 그리고 어떻게 하면 더 효율적으로 사용할 수 있는지 탐구해 봅시다.
5.1 대용량 로그 파일 분석
대용량 로그 파일을 분석하는 것은 Stream API의 강점을 잘 보여주는 사례입니다.
public class LogAnalyzer {
public static Map<String, Long> analyzeLogFile(String filePath) throws IOException {
try (Stream<String> lines = Files.lines(Paths.get(filePath))) {
return lines
.filter(line -> line.contains("ERROR"))
.map(line -> line.split(" ")[2]) // 에러 코드 추출
.collect(Collectors.groupingBy(
Function.identity(),
Collectors.counting()
));
}
}
}
// 사용 예
Map<String, Long> errorCounts = LogAnalyzer.analyzeLogFile("server.log");
errorCounts.forEach((code, count) ->
System.out.println("Error code " + code + ": " + count + " occurrences"));
이 예제는 대용량 로그 파일을 스트림으로 읽어 에러 코드별 발생 횟수를 계산합니다. 파일을 한 번에 메모리에 로드하지 않고 라인 단위로 처리하므로 메모리 효율적입니다.
5.2 복잡한 데이터 변환 및 집계
금융 데이터를 처리하는 시나리오를 가정해 봅시다. 여러 계좌의 거래 내역을 분석하고 요약하는 작업을 Stream API로 구현할 수 있습니다.
class Transaction {
String accountId;
LocalDate date;
double amount;
// 생성자, getter 생략
}
public class FinancialAnalyzer {
public static Map<String, DoubleSummaryStatistics> analyzeTransactions(List<Transaction> transactions) {
return transactions.stream()
.collect(Collectors.groupingBy(
Transaction::getAccountId,
Collectors.summarizingDouble(Transaction::getAmount)
));
}
}
// 사용 예
List<Transaction> transactions = // 거래 내역 리스트
Map<String, DoubleSummaryStatistics> accountSummaries = FinancialAnalyzer.analyzeTransactions(transactions);
accountSummaries.forEach((accountId, stats) -> {
System.out.println("Account: " + accountId);
System.out.println(" Total: " + stats.getSum());
System.out.println(" Average: " + stats.getAverage());
System.out.println(" Max: " + stats.getMax());
System.out.println(" Min: " + stats.getMin());
});
이 예제는 각 계좌별로 거래 금액의 합계, 평균, 최대, 최소값을 한 번의 스트림 연산으로 계산합니다.
5.3 동적 필터링 및 정렬
사용자의 입력에 따라 동적으로 필터링 및 정렬 조건을 적용하는 시나리오를 살펴봅시다.
class Product {
String name;
double price;
String category;
// 생성자, getter 생략
}
public class ProductFilter {
public static List<Product> filterAndSort(List<Product> products,
String category,
Double minPrice,
Double maxPrice,
String sortBy) {
Stream<Product> stream = products.stream();
if (category != null) {
stream = stream.filter(p -> p.getCategory().equals(category));
}
if (minPrice != null) {
stream = stream.filter(p -> p.getPrice() >= minPrice);
}
if (maxPrice != null) {
stream = stream.filter(p -> p.getPrice() <= maxPrice);
}
if ("name".equals(sortBy)) {
stream = stream.sorted(Comparator.comparing(Product::getName));
} else if ("price".equals(sortBy)) {
stream = stream.sorted(Comparator.comparingDouble(Product::getPrice));
}
return stream.collect(Collectors.toList());
}
}
// 사용 예
List<Product> products = // 상품 리스트
List<Product> filtered = ProductFilter.filterAndSort(products, "electronics", 100.0, 500.0, "price");
이 예제는 사용자의 입력에 따라 동적으로 필터와 정렬 조건을 적용합니다. Stream API의 유연성을 잘 보여주는 사례입니다.
5.4 병렬 처리를 통한 성능 최적화
대용량 데이터셋에 대해 복잡한 연산을 수행할 때, 병렬 스트림을 활용하여 성능을 크게 향상시킬 수 있습니다.
public class PrimeCalculator {
public static long countPrimes(int upTo) {
return IntStream.range(2, upTo)
.parallel()
.filter(PrimeCalculator::isPrime)
.count();
}
private static boolean isPrime(int number) {
return IntStream.rangeClosed(2, (int) Math.sqrt(number))
.noneMatch(i -> number % i == 0);
}
}
// 사용 예
long start = System.currentTimeMillis();
long primeCount = PrimeCalculator.countPrimes(1_000_000);
long end = System.currentTimeMillis();
System.out.println("Found " + primeCount + " primes");
System.out.println("Time taken: " + (end - start) + "ms");
이 예제는 주어진 범위 내의 소수 개수를 병렬로 계산합니다. 병렬 스트림을 사용함으로써 멀티코어 프로세서의 이점을 활용할 수 있습니다.
5.5 커스텀 컬렉터를 통한 복잡한 집계
때로는 기본 제공되는 컬렉터로는 부족할 때가 있습니다. 이런 경우 커스텀 컬렉터를 만들어 사용할 수 있습니다.
class Order {
String customerId;
double amount;
// 생성자, getter 생략
}
public class OrderAnalyzer {
public static Map<String, CustomerStats> analyzeOrders(Stream<Order> orders) {
return orders.collect(Collectors.groupingBy(
Order::getCustomerId,
Collector.of(
CustomerStats::new,
CustomerStats::accumulate,
CustomerStats::combine
)
));
}
}
class CustomerStats {
int orderCount = 0;
double totalAmount = 0;
double maxAmount = Double.MIN_VALUE;
double minAmount = Double.MAX_VALUE;
void accumulate(Order order) {
orderCount++;
totalAmount += order.getAmount();
maxAmount = Math.max(maxAmount, order.getAmount());
minAmount = Math.min(minAmount, order.getAmount());
}
CustomerStats combine(CustomerStats other) {
orderCount += other.orderCount;
totalAmount += other.totalAmount;
maxAmount = Math.max(maxAmount, other.maxAmount);
minAmount = Math.min(minAmount, other.minAmount);
return this;
}
}
// 사용 예
Stream<Order> orders = // 주문 스트림
Map<String, CustomerStats> customerStats = OrderAnalyzer.analyzeOrders(orders);
customerStats.forEach((customerId, stats) -> {
System.out.println("Customer: " + customerId);
System.out.println(" Order Count: " + stats.orderCount);
System.out.println(" Total Amount: " + stats.totalAmount);
System.out.println(" Max Amount: " + stats.maxAmount);
System.out.println(" Min Amount: " + stats.minAmount);
});
이 예제는 커스텀 컬렉터를 사용하여 고객별 주문 통계를 계산합니다. 이를 통해 복잡한 집계 로직을 효율적으로 구현할 수 있습니다.
5.6 무한 스트림을 활용한 데이터 생성
무한 스트림을 활용하여 복잡한 데이터 시퀀스를 생성할 수 있습니다.
public class FibonacciGenerator {
public static Stream<BigInteger> fibonacci() {
return Stream.generate(new Supplier<BigInteger[]>() {
BigInteger[] arr = new BigInteger[]{BigInteger.ZERO, BigInteger.ONE};
public BigInteger[] get() {
BigInteger[] current = arr.clone();
arr[0 ] = arr[1];
arr[1] = arr[0].add(arr[1]);
return current;
}
}).map(arr -> arr[0]);
}
}
// 사용 예
FibonacciGenerator.fibonacci()
.limit(100)
.forEach(System.out::println);
이 예제는 무한한 피보나치 수열을 생성합니다. limit()
를 사용하여 원하는 만큼만 생성할 수 있습니다.
5.7 스트림 결과 캐싱
동일한 스트림 연산을 여러 번 수행해야 할 경우, 결과를 캐싱하여 성능을 향상시킬 수 있습니다.
public class ExpensiveOperation {
private static Map<Integer, Integer> cache = new ConcurrentHashMap<>();
public static int compute(int input) {
return cache.computeIfAbsent(input, i -> {
// 복잡한 계산 시뮬레이션
try {
Thread.sleep(1000);
} catch (InterruptedException e) {
Thread.currentThread().interrupt();
}
return i * i;
});
}
}
// 사용 예
List<Integer> inputs = Arrays.asList(1, 2, 3, 4, 5, 1, 2, 3, 4, 5);
long start = System.currentTimeMillis();
List<Integer> results = inputs.stream()
.map(ExpensiveOperation::compute)
.collect(Collectors.toList());
long end = System.currentTimeMillis();
System.out.println("Results: " + results);
System.out.println("Time taken: " + (end - start) + "ms");
이 예제는 비용이 많이 드는 연산의 결과를 캐싱하여, 동일한 입력에 대해 반복 계산을 피합니다.
5.8 스트림 디버깅 최적화
복잡한 스트림 연산을 디버깅할 때, 로깅을 효율적으로 사용할 수 있습니다.
public class StreamDebugger {
private static <T> Consumer<T> peek(Consumer<T> action) {
return t -> {
action.accept(t);
return t;
};
}
public static void main(String[] args) {
List<Integer> numbers = Arrays.asList(1, 2, 3, 4, 5, 6, 7, 8, 9, 10);
List<Integer> result = numbers.stream()
.filter(peek(n -> System.out.println("Filtering: " + n)))
.map(peek(n -> System.out.println("Mapping: " + n)))
.limit(5)
.collect(Collectors.toList());
System.out.println("Final result: " + result);
}
}
이 예제는 각 단계에서 요소의 상태를 로깅하여 스트림 연산의 흐름을 쉽게 파악할 수 있게 합니다.
5.9 스트림 분할 처리
대용량 데이터를 처리할 때, 스트림을 분할하여 처리하면 메모리 사용을 최적화할 수 있습니다.
public class LargeDataProcessor {
public static void processLargeData(String filePath, int batchSize) throws IOException {
try (BufferedReader reader = new BufferedReader(new FileReader(filePath))) {
Stream<String> lines = reader.lines();
Iterator<String> iterator = lines.iterator();
while (iterator.hasNext()) {
List<String> batch = new ArrayList<>(batchSize);
for (int i = 0; i < batchSize && iterator.hasNext(); i++) {
batch.add(iterator.next());
}
processBatch(batch);
}
}
}
private static void processBatch(List<String> batch) {
// 배치 처리 로직
batch.stream()
.map(String::toUpperCase)
.forEach(System.out::println);
}
}
// 사용 예
LargeDataProcessor.processLargeData("very_large_file.txt", 1000);
이 예제는 대용량 파일을 일정 크기의 배치로 나누어 처리함으로써 메모리 사용을 제어합니다.
5.10 복잡한 집계 연산 최적화
여러 가지 집계 연산을 한 번에 수행해야 할 때, 커스텀 컬렉터를 사용하여 효율성을 높일 수 있습니다.
class SalesRecord {
String product;
double amount;
// 생성자, getter 생략
}
class SalesStats {
double totalSales = 0;
int count = 0;
double maxSale = Double.MIN_VALUE;
String bestSellingProduct = "";
void accumulate(SalesRecord record) {
totalSales += record.getAmount();
count++;
if (record.getAmount() > maxSale) {
maxSale = record.getAmount();
bestSellingProduct = record.getProduct();
}
}
SalesStats combine(SalesStats other) {
totalSales += other.totalSales;
count += other.count;
if (other.maxSale > maxSale) {
maxSale = other.maxSale;
bestSellingProduct = other.bestSellingProduct;
}
return this;
}
}
public class SalesAnalyzer {
public static SalesStats analyzeSales(Stream<SalesRecord> sales) {
return sales.collect(Collector.of(
SalesStats::new,
SalesStats::accumulate,
SalesStats::combine
));
}
}
// 사용 예
Stream<SalesRecord> salesStream = // 판매 기록 스트림
SalesStats stats = SalesAnalyzer.analyzeSales(salesStream);
System.out.println("Total Sales: " + stats.totalSales);
System.out.println("Number of Sales: " + stats.count);
System.out.println("Best Selling Product: " + stats.bestSellingProduct);
System.out.println("Highest Sale Amount: " + stats.maxSale);
이 예제는 판매 데이터에 대해 여러 가지 통계를 한 번의 스트림 연산으로 계산합니다.
이러한 고급 활용 사례와 최적화 기법들은 Stream API의 강력함과 유연성을 잘 보여줍니다. 재능넷에서 Java 프로그래밍 지식을 공유할 때, 이런 실전적이고 심화된 내용은 독자들의 기술적 성장에 큰 도움이 될 것입니다.
Stream API를 마스터하면 더 효율적이고 읽기 쉬운 코드를 작성할 수 있으며, 복잡한 데이터 처리 작업을 우아하게 해결할 수 있습니다. 계속해서 실험하고 학습하면서 Stream API의 잠재력을 최대한 활용해 보세요! 🚀